Tips on how to ‘unlock’ your data analysis
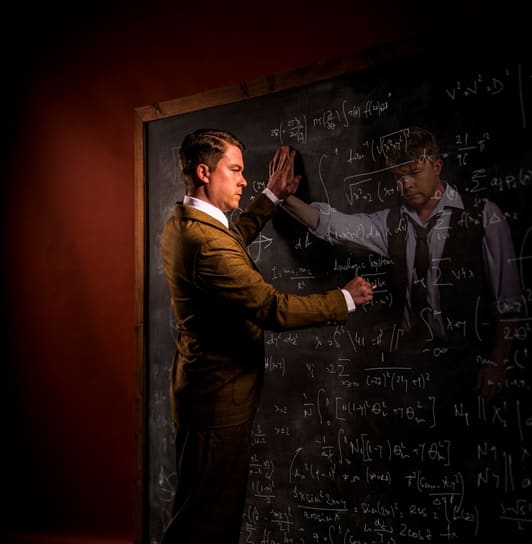
A common problem with analysing qualitative data, which both students and more experienced researchers face, is… simply getting stuck with it. It’s like writer’s block, but in data analysis – you may have all the necessary components in theory, but you are not sure what to do with them. You are not sure what to analyse and how, where to look, what to focus on, etc. Here are some steps that always help me “unlock” my data analysis and dig deeper into the data (the order is random, you may apply some, or all of them, and at various stages of your work):
- Read through the data, without worrying about coding. Try to get the overall “feel” of it, occasionally recording some thoughts that may occur (e.g. if you see patterns across the data, some similarities/differences, something that you think could turn out to be significant, etc.)
- Try to sketch a simple model of what you see in the data. You can either do it with a computer software (data analysis software, such as NVivo, or specific software for designing models – I have used https://www.draw.io/) or by hand. I quite often do it on a separate piece of paper, which results in hundreds of pages with weird and difficult to interpret “hieroglyphics” scattered around my house. In the model, try to “theorize” about your data, potential links, relationships and associations between different elements of your project (e.g. the themes you develop, or certain experiences/beliefs described by your participants), possible causal relationships (e.g. this seems to result in that) and other things you notice. This is supposed to be theoretical, so don’t be afraid to make assumptions and jump into conclusions – this is Exactly what you need. As a result, you have a whole bunch of possible relationships, assumptions and “hunches” to investigate and check if they are, in fact, true.
- Write about your data (you can do it as a free writing exercise) – again, as with the model, write about what you have found, as well as about what you think you may find, write about everything that seems to be relevant in any way. Similar to drawing the model, this may help you “open up” your data, come up with ideas that you may later explore and investigate (and this will be the actual analysis part of your data analysis)
- Run word frequency queries – to see which words appear most frequently in your data. This sometimes helps to draw your attention to issues that you previously did not pay attention to
- “Dig deep” into what the content of what the participants said meant to them – go beyond simple factual information that they convey, try to see who said something, how they said it, under what circumstances, when they decided to talk about it (e.g. which interview questions prompted them to give a certain response), see what followed these thoughts, what they chose to speak about (e.g. do they always talk about stressful experiences at school when you ask them about their childhood?), what kind of emotions they seem to experience when discussing particular topics, etc.
- Conduct a detailed “within-case analysis” – focus on each participant individually, considering some of the points made above, as well as whether one participant changed his/her opinion within the same interview, how he/she reacted to similar questions asked at different stages in the interview, etc. If you have a coding framework, analyse it in detail for one participant at the time. Try to draw a model for each participant. Write a case summary – summarize what a given participant said, what your thoughts and observations based on this are, etc. If you have more than one data set for an individual participant (e.g. an interview transcript, a diary, open-ended questionnaire responses, etc.), treat them as one “case” and explore all these data sets in detail.
- Conduct a detailed “cross-case analysis” – follow similar steps but this time with the aim of comparing the “cases” (e.g. different participants), looking for similarities and differences, comparing these similarities/differences in relation to (demographic) characteristics of the participants (e.g. did those with more teaching experience express more positive attitudes towards something than those with no experience, did men express similar opinions to women, did students share similar views to their teachers, etc.)
- If you have a coding framework – create matrices that compare the coding between the participants in relation to the above kind of characteristics (in NVivo, this is called a matrix coding query)
- Check whether the participants repeat similar points, whether there are re-occurring patterns, when these patterns occur
- Investigate “negative cases” – these are the cases (e.g. participants) that, for any reason, do not “fit” the rest of cases or your emerging “theory” or explanation (e.g. what one single participant said is completely the opposite to what all, or the vast majority of, the others said). I love investigating negative cases – remember that they don’t “ruin” your theory, or your analysis in general, but rather enrich it and provide a lot of new insights
Do you have any other tips for “unlocking” your data? Please share them in the comments!